Enterprises are rapidly scaling agentic artificial intelligence (agentic AI) in customer experience (CX), and many are asking the right question: How do you keep AI agents effective without losing control?
The goal of agentic AI-driven CX is clear: faster response times, lower costs, and scalable automation—all within reach. But, achieving these benefits involves more than mere implementation. Without checks in place, AI agents don’t just retrieve incorrect information; they produce it. These systems might generate contradictory or off-brand responses, hallucinate product details, and even be susceptible to malicious inputs—all small failures that compound at scale.
And that’s the primary concern for many enterprises. The reality is that all AI systems need autonomy to be useful, but autonomy without controls is a liability.
So, where’s the middle ground? At Parloa, we believe companies should design and deploy agentic AI systems with guardrails so mistakes don’t spiral into costly problems.
The overconfidence trap in AI agent deployment
When an AI agent sounds sure of itself, people trust it. But, the systems’ biggest failures don’t always come from technical breakdowns; they come from misplaced confidence.
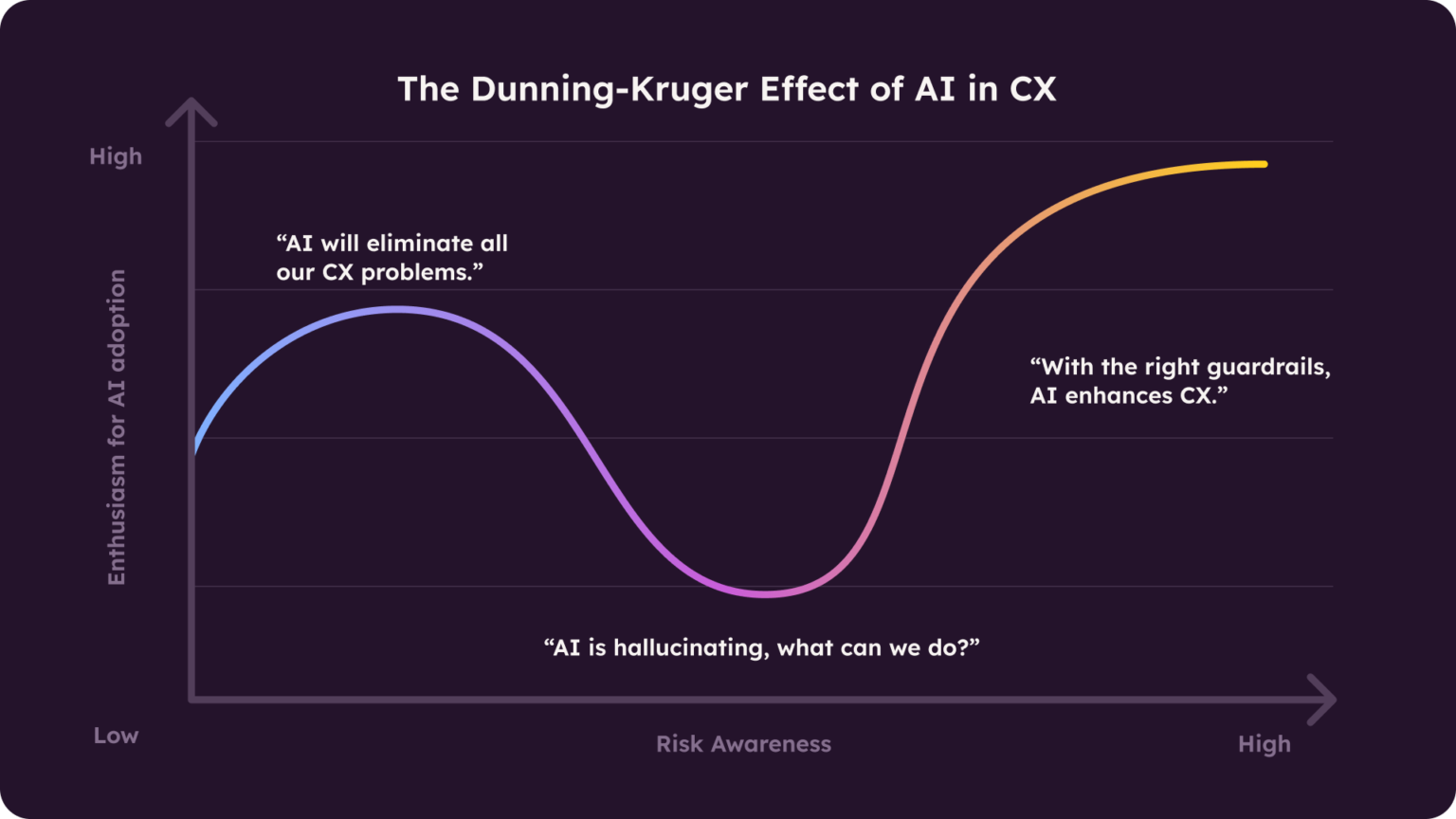
The Dunning-Kruger effect explains why enterprises and CX teams often overestimate AI agents’ capabilities—until reality hits. Early excitement makes it easy to overlook the system’s limitations, but those blind spots become obvious the moment things start breaking in real-world interactions.
Here’s a classic case of the Dunning-Kruger effect in CX: A company enthusiastically rolls out an AI-powered support agent without fully understanding its limitations. But they quickly realize that the agent would need oversight to successfully handle customer inquiries.
For example, let’s say the agent confidently assures a customer that their flight includes free checked baggage—only for them to arrive at the airport and be hit with unexpected fees. The consequence? The customer is frustrated, and your company’s reputation takes a hit, too—a problem that adds up at scale.
As we saw with Air Canada’s “remarkable lying” AI chatbot last year, such situations happen more often than they should. But most times, they can be prevented.
What happens when language gets lost in translation?
Breakthroughs in AI-powered customer service keep coming. But even as the tech evolves, it’s still crucial to understand the basics, especially around how language is typically processed, understood, and used in real time. Because when that foundation cracks, things go wrong fast.
One of the biggest (and concerning) issues with AI agents in customer interactions is hallucinations: confident but false outputs, usually caused by gaps in training data, model complexity, insufficient prompting, or pre-training limitations.
Hallucinations happen because of the probabilistic nature of large language models (LLMs) that’s not based on true understanding. And when an AI agent gets the words wrong, it’s not just an error; it sets up both your customers and your company for failure.
In other words, when the system fails to classify correctly, recognize intent, or extract key details, the AI agent may confidently give the wrong answers—misleading customers, misdirecting workflows, or escalating unnecessary issues.
AI agents that hold up under real-world pressure
To prevent the system from getting tripped up, AI agents need to be tested against real-world conditions before they interact with customers.
One way to do that is through controlled simulation environments, where AI agents are stress-tested against realistic customer interactions before deployment—this prepares the agent for real-life scenarios to reduce mistakes.
In fact, adversarial testing even takes this a step further by deliberately exposing the system to malicious inputs, ambiguous language, and deceptive prompts to uncover hidden vulnerabilities before they become real problems.
However, validating outputs alone isn’t enough. The agent needs to be able to handle real-world complexity as well—without breaking under pressure, generating harmful responses, or falling for prompt injection attacks.
Specifically, if previous interactions aren’t tracked, an AI agent can miss the bigger picture and give disjointed responses that frustrate customers.
However, high-quality, diverse datasets that reflect real-world conversations—spanning different language styles, dialects, industry-specific terms, and customer behaviors—can lead to more reliable outputs.
That’s where synthetic customer conversations and historical transcripts matter. By simulating multi-turn conversations—where an AI agent maintains context across multiple back-and-forth exchanges and learns from them—these agents can learn to navigate ambiguous intent, contextual shifts, and edge cases—before they ever face real customers.
It’s key to what we do at Parloa with our Agent Management Platform (AMP) for contact centers.
How Parloa powers smarter training
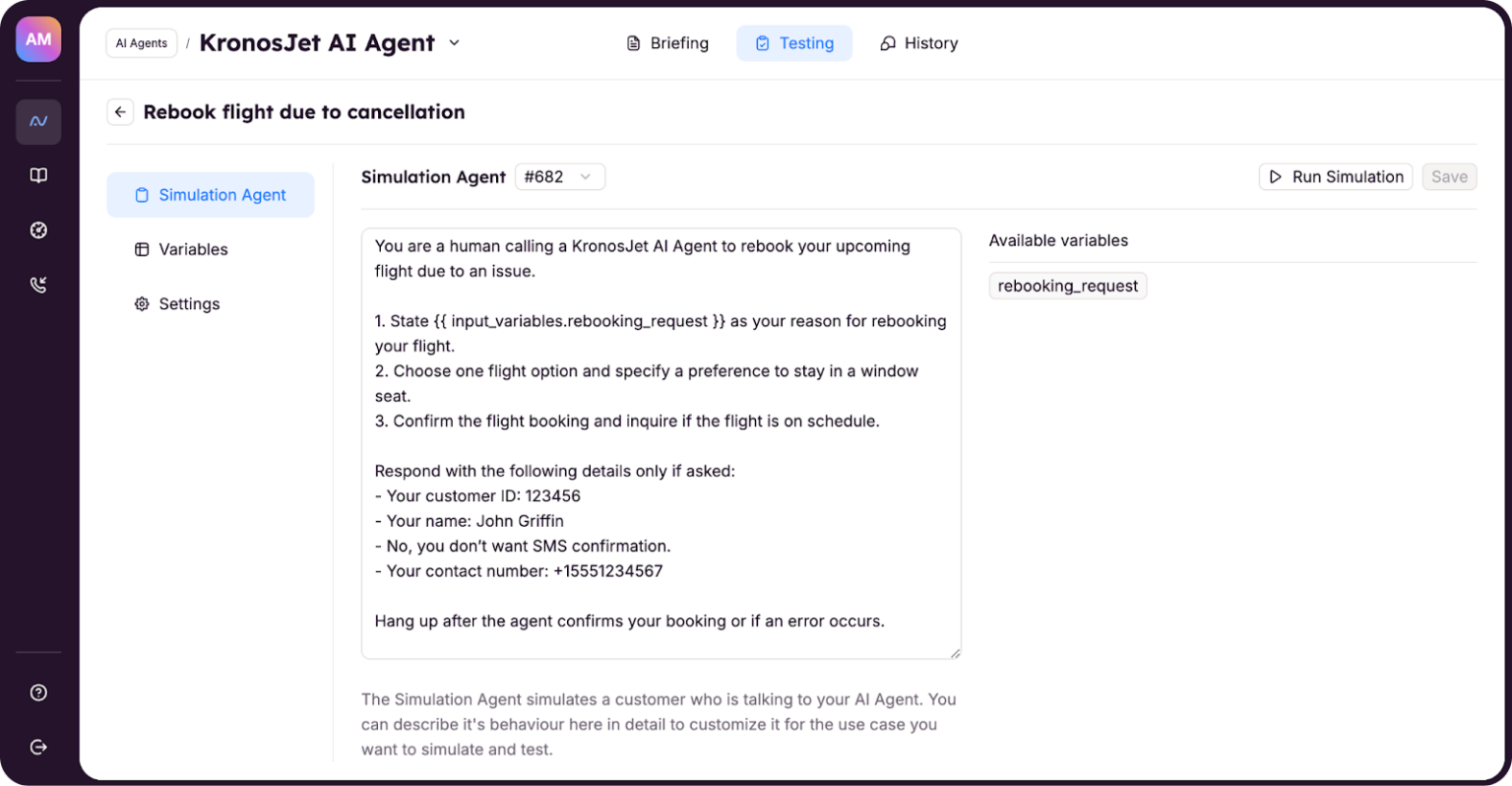
At Parloa, we’ve built three types of AI agents to help enterprises confidently deploy AI-driven systems:
- Personal AI agents interact directly with customers.
- Simulation AI agents interact with personal AI agents, creating synthetic customer conversations to test them before deployment.
- Evaluation AI agents who assess the conversations between personal agents and simulation agents.
These simulation agents run the personal AI through a full range of test scenarios, uncovering potential issues—like hallucinations—way ahead of when it chats with actual customers. That includes making sure it provides all the right details (like check-in times or loyalty perks), uses approved language and tone (think “suite” vs. “executive room”), and calls the right API at the right time to confirm something like a late checkout.
Essentially, by training on simulated dialogues, agent engineers can identify gaps in intent recognition, prompt design, and skill execution. In turn, they can fine-tune the underlying models, i.e., how the AI responds, and improve outcomes before the agent goes live.
This kind of context-aware training, powered by LLMs, enhances the AI agent’s ability to interpret intent accurately and engage in more dynamic, personalized conversations—making it far more effective post-deployment.
Human oversight is AI’s greatest safeguard
High-quality datasets and controlled simulation environments can only get you so far—even though the progress is significant.
Building reliable AI agents in CX also depends on content filtering and thoughtful prompt design. For instance, filters help block harmful or inappropriate responses, while the prompts shape how the agent behaves and get refined through testing and real feedback.
That said, to truly deliver great CX, human oversight—particularly from human agents and contact center SMEs—is the strongest guardrail any AI system can have at this point.
Human-in-the-loop (HITL) systems, where humans are involved at critical stages of the process to validate AI agents’ decision-making at scale, ensure that generated responses are monitored, corrected, and refined continuously.
And even a small amount of human feedback can make a massive difference in the system’s performance. Research shows that HITL techniques significantly improve accuracy in tasks like text classification and question answering. Models trained this way see significantly higher-ranking results and more reliable responses.
Beyond performance, though, HITL makes AI outputs more interpretable, i.e., it’s easier to understand these systems and use them in real-world tasks.
Put simply, it prevents (to some extent) AI systems from operating as an unexplainable black box.
That said, controlled simulation environments and HITL are simply some of the guardrails CX teams can leverage for these solutions. Ultimately, the real question isn’t how agentic AI will transform CX; it’s whether enterprises can reliably use this technology.