As generative AI transforms customer interactions, companies must rethink their approach to managing AI-powered automation. This blog explores key strategies for effective deployment, ongoing lifecycle management, and risk mitigation during deployment and beyond.
The old playbook for customer experience was clear: enhance scripted dialog flows with tools like natural language processing, intent classification, and rule-based workflows. This approach addressed challenges such as call deflection and became a widely adopted best practice among organizations.
But the game has changed. Generative AI entered the stage and we moved beyond enhancing predefined conversation to creating autonomous, personalized AI agents capable of handling complex tasks independently. With this leap, however, comes an array of new challenges: issues with tool calling, hallucinations, and the risk of the AI Agent going off track from their intended behavior.
When these challenges are multiplied across millions of users — each with their own personalized AI agent — the scale of deployment and oversight becomes exponentially more daunting. For example, 10 million customers, that’s not just 10 million conversations to oversee, but also 10 million individual agents to manage safely and effectively.
The stakes couldn’t be higher. To succeed, organizations need more than just a one-time implementation plan; they must have a clear strategy for both the deployment and ongoing lifecycle management of these agents.This is where the new rules of AI Agent management come into play.
Breaking It Down: 4 Steps to Safely and Effectively Manage AI Agents
Implementing an AI system involves many moving parts — agent skills, human-in-the-loop integration, data and model orchestration, and, most importantly, agent lifecycle management.
Lifecycle management is the foundation that ensures agents function properly, stay safe, and continuously improve, influencing every other part of the system. This process can be broken into four key steps: designing and integrating, simulating and evaluating, deploying and scaling, as well as monitoring and improving AI agents. Together, these steps create a continuous cycle that ensures reliability while driving ongoing learning and improvement over time.
1. Design & Integrate
The first step in managing AI agents is to define their goals and constraints while connecting them to existing data and systems. This stage marks an important shift from traditional programming to briefing agents through natural language, enabling faster and more flexible deployment. It involves setting the agent’s role, tone, instructions, goals, and the skills needed to achieve those goals. For example, enabling user authentication requires the agent to connect with third-party systems like CRMs or ERPs via APIs to fetch data and dispatch actions. Since these external systems, which enable the agents skills, are not controlled by the AI platform, such integrations can introduce issues down the line like disrupting the agent’s functionality — underscoring the importance of proper lifecycle management.
2. Simulate & Evaluate
The second step in managing AI agents focuses on ensuring quality and reliability through automated simulation and evaluation. This stage is essential for adapting to the shift from a deterministic world – where decision-making follows clearly defined paths — to a non-deterministic one, where large language models (LLMs) act dynamically based on input. This flexibility brings risks, such as agents going off track and failing to meet their goals.
To mitigate these risks, AI agents, their prompts, and integrations must undergo rigorous testing using hundreds or thousands of simulated conversations. Automated simulations are key to detecting misconfigurations and ensuring reliability at scale. Effective simulation and evaluation require leveraging real customer interactions to design realistic simulated behaviors, all generated through natural language rather than code or flow-based logic. This includes testing scenarios involving cooperative, uncooperative, or frustrated customers.
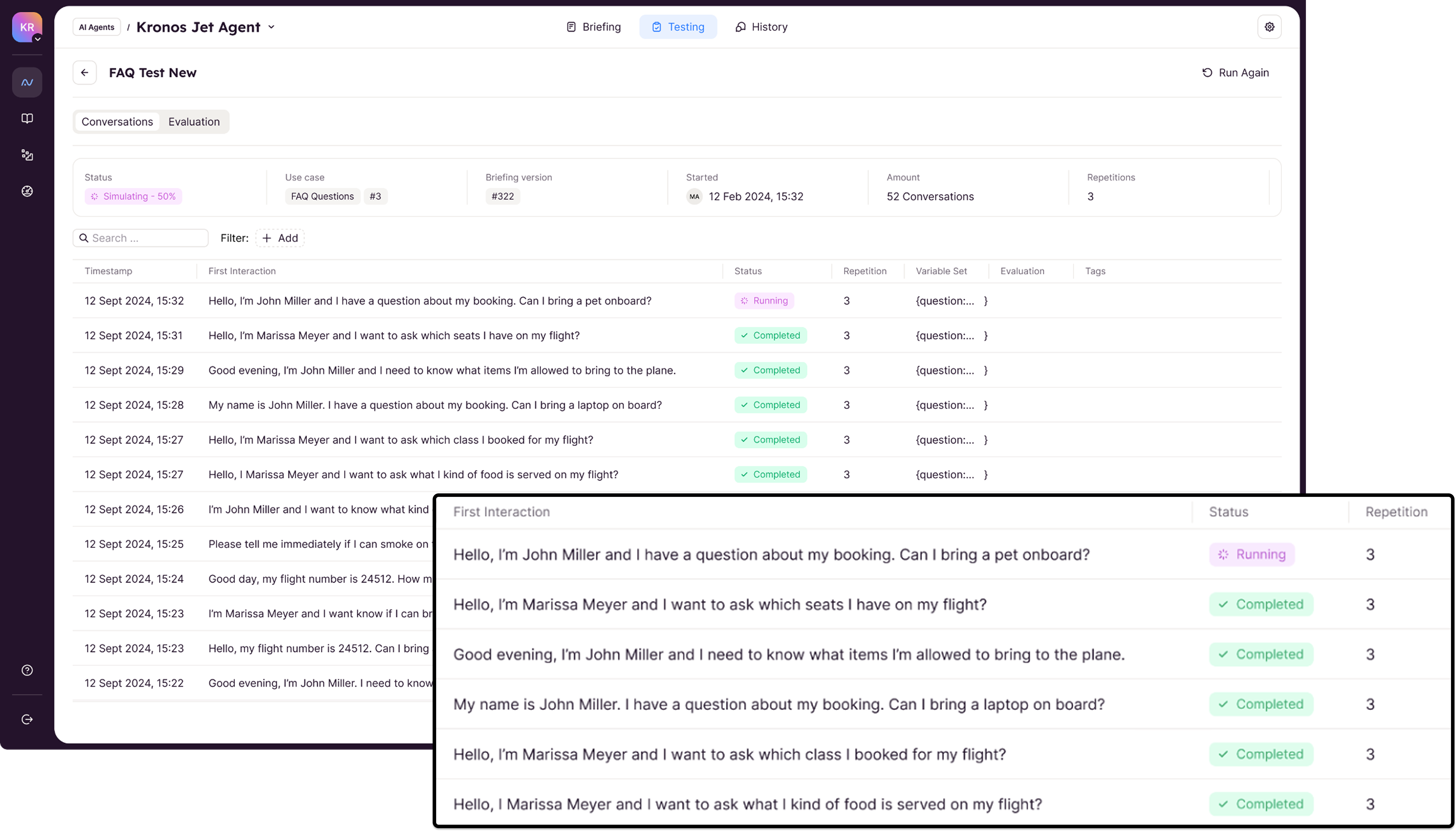
Evaluation is then carried out by another AI agent, programmed to assess whether conversations meet predefined success criteria. These criteria can include technical factors like API or JSON errors and conversational milestones. The insights generated by this evaluation process guide human AI managers in making necessary adjustments. With these simulated customers and evaluators in place, companies can continuously test agents after updates, avoiding issues like conflicting information or unintended actions.
3. Deploy & Scale
The third step in managing AI agents is launching versioned agents and rolling them out across customer conversations. This involves connecting agents to the respective environments, starting with staging for testing and then moving to production. A version log is maintained to ensure that, if any issues arise, a safe and tested previous version can be quickly redeployed — an essential feature for an enterprise-ready solution.
Core to deployment is configuring essential infrastructure, such as VOIP settings, which include phone numbers and ZIP integrations. With systems like our AI Agent Management Platform (AMP), deploying agents is streamlined and can be done instantly with the press of a button. Additionally, the inbound call infrastructure automatically scales in the background, adapting to fluctuating call volumes without manual intervention.
4. Monitor & Improve
The final step in managing AI agents is tracking their performance and refining their behavior based on real-world insights. This process involves converting conversations into data and then analyzing it to generate actionable insights.
Performance indicators are selected to measure success, often mirroring traditional contact center metrics such as resolution time and handover rates. This step is integral to the continuous improvement cycle, enabling teams to optimize agents based on specific priorities, such as reducing handover rates rather than focusing solely on resolution times.
By analyzing data, teams can improve prompts, refine data sources, and identify gaps in agent capabilities. For instance, a high handover rate might indicate the need to develop new skills for the agent to handle previously escalated tasks. This iterative approach ensures that agents not only maintain performance but continuously evolve to meet customer needs more effectively.
AI Agent Management: A New Playbook for Safe and Effective Deployment
The shift from scripted dialog flows to autonomous AI agents brings both opportunities and challenges. Traditional approaches focused on enhancing interactions with NLP and intent classification, but generative AI now enables personalized agents that handle complex tasks independently. Managing these agents at scale requires a structured approach.
AI agent lifecycle management follows four key steps:
- Design & Integrate – Define agent roles, goals, and system integrations.
- Simulate & Evaluate – Test agent reliability through automated simulations.
- Deploy & Scale – Roll out versioned agents with controlled infrastructure.
- Monitor & Improve – Continuously analyze performance and refine behavior.
A strategic approach not only ensures that AI agents remain reliable, effective, and aligned with business goals but also paves the way for future innovation, empowering organizations to stay ahead of the curve in an ever-evolving landscape of AI-driven customer experiences.